Hill-Making vs Hill-Climbing
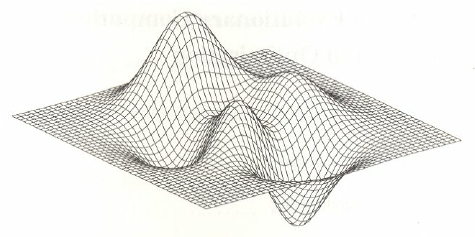
There are two modes of learning, two paths to improvement. One is to relentlessly, deliberately improve what you can do already, by trying to perfect your process. Focus on optimizing what works. The other way is to create new areas that can be exploited and perfected. Explore regions that are suboptimal with a hope you can make them work – and sometimes they will – giving you new territory to work in. Most attempts to get better are a mix of these methods, but in their extremes these two functions – exploit and explore – operate in different ways, and require different strategies.
The first mode, exploiting and perfecting, rewards efficiency and optimization. It has diminishing returns, becoming more difficult as fitness and perfection is increased. But it is reliable and low risk. The second mode, exploring and creating, on the other hand, is highly uncertain, with high risks, yet there is less competition in this mode and the yields by this approach are, in theory, unlimited.
This trade off between exploiting and exploring is present in all domains, from the personal to the biggest systems. Balancing the desire to improve your own skills versus being less productive while you learn new skills is one example at the personal level. The tradeoff between investing heavily in optimizing your production methods instead of investing in new technology that will obsolete your current methods is an example of that tradeoff at the systems level. This particular tradeoff in the corporate world is sometimes called the “innovator’s dilemma.” The more efficient and excellent a company becomes, the less it can afford to dabble in some new-fangled idea that is very likely to waste investments that can more profitably be used to maximize their strengths. Since statistically most new inventions will fail, and most experiments will not pan out, this reluctance for the unknown is valid.
More importantly, if new methods do succeed, they will cannibalize the currently optimal products and processes. This dilemma makes it very hard to invest and explore new products, when it is far safer, more profitable, to optimize what already works. Toyota has spent many decades optimizing small efficient gasoline combustion engines; no one in the world is better. They are at the peak of gas car engines. Any money spent on investing into unproven alternative engines, such as electric motors, would reduce their profits. They would be devolving their expertise and becoming less excellent. They cannot afford to be less profitable.
Successful artists, musicians and other creatives have a similar dilemma. Their fans want more of what they did to reach their success. They want them to play their old songs, paint the same style paintings, and make the same kind of great movies. But in order to keep improving, to maintain their reputation as someone creative, the artists need to try new stuff, which means they will make stuff that fails, that is almost crap, that is not excellent. To win future fans the artist has to explore rather than merely exploit. They have to take up a new instrument, or develop a new style, or invent new characters, which is risky. Sequels are just so much more profitable.
But even sequels – if they are to be perfect – are not easy. They take a kind of creativity to perfect. This kind of everyday creativity, the kind of problem solving that any decent art or innovation requires, is what we might call “lower-case” or base creativity. Devising a new logo, if done well, requires creativity. But designing logos is a well-trod domain, with hundreds of thousands of examples, with base creativity as the main ingredient. Designing another great book cover is primarily a matter of exploiting known processes and skills. Occasionally someone will step up and create a book cover so unusual and weird but cool that it creates a whole new way to make covers in the future. This is upper-case, disruptive Creativity.
Upper-case, disruptive Creativity discovers or creates new areas to be creative in. It alters the landscape. Most everyday base creativity is filling in the landscape rather than birthing it. Disruptive Creativity is like the discovery of DNA, or the invention of photography, or Impressionism in art. Rather than just solving for the best possibility, it is enlarging the space of all possibilities.
Both biologists and computer scientists use the same analogy when visualizing this inherent trade-off between optimization and exploration. Imagine a geological landscape with mountains and valleys. The elevation of a place on the landscape is reckoned as its fitness, or its technical perfection. The higher up, the more fit. If an organism, or a product, or an answer is as perfect as it can be, then it registers as being at the very peak of a mountain, because it cannot be any more fit or perfect for its environment. Evolution is pictured as the journey of an organism in this conceptual landscape. Over time, as the organism’s form adapts more and more to its environment, this change is represented as that organism going up (getting better), as it climbs toward a peak. In shorthand, the organism is said to be hill-climbing.
Computer scientists employ the same analog to illustrate how an algorithm can produce the best answer. If higher up in the landscape represents better answers then every slope has a gradient. If your program always rewards any answer higher up the gradient, then over time it will climb the hill, eventually reaching the optimal peak. The idea is that you don’t really have to know what direction to go, as long as you keep moving up. Computer science is filled with shortcuts and all kinds of tricks to speed up this hill-climbing.
In the broadest sense, most every-day creativity, ordinary innovation, and even biological adaptation in evolution is hill climbing. Most new things, new ideas, most improvements are incremental advances, and for most of the time, this optimization is exactly what is needed.
But every now and then an upper-case, disruptive jump occurs, creating a whole new genre, a new territory, or a new way to improve. Instead of incrementally climbing up the gradient, this change is creating a whole new hill to climb. This process is known as hill-making rather than hill climbing.
Hill-making is much harder to do, but far more productive. The difficulty stems from the challenge of finding a territory that is different, yet plausible, inhabitable, coherent, rather than just different and chaotic, untenable, or random nonsense. It is very easy to make drastic change, but most drastic changes do not work. Hill-making entails occupying (or finding*) an area where your work increases the possibilities for more work.
If a musician invents a new instrument, rather than just another song, that new instrument opens up a whole new area to write many new songs in, new ways to make music that could be exploited and explored by many. Inventing the technology of cameras was more than merely an incremental step in optics, it opened up vast realms of visual possibilities, each of those, such as cinema, or photojournalism, became new hills that can be climbed. The first comedians to figure out stand-up comedy, created a whole new hill – a new vocation that could be perfected.
The techniques needed for this upper-case Creativity, this disruptive innovation, this hill-making, are significantly different from the techniques for hill-climbing. To date, generative LLM-AI is capable of generating lower-case base creativity. It can create novel images, novel text, novel solutions that are predominately incremental. These products may not be things we’ve seen or heard before, or even things we would have thought of ourselves, but on average the LLMs do not seem to be creating entirely new ways to approach text, images, and tasks. So far they seem to like the skill of making a new hill.
Hill-making demands huge inefficiencies. There is a lot of wandering around, exploring, with plenty of dead ends, detours, and dry deserts where things die. The cost of exploring is that you only discover something worthwhile occasionally. This is why the kinds of paths that tend toward hill-making such as science, entrepreneurship, art, and frontier exploration are inherently expensive. Most of the time nothing radically new is discovered. Not only is patience needed, but hill-making requires a high tolerance of failure. Wastage is the norm.
At the same time, hill-finding requires the ability to look way beyond current success, current fitness, current peaks. Hill-finding cannot be focused on “going up the gradient” – becoming excellent. In fact it requires going down the gradient, becoming less excellent, and in a certain sense, devolving. The kind of agents that are good at evolving towards excellence are usually horrible in devolving toward possible death. But the only way to reach a new hill – a hill that might potentially grow to be taller than the hill you are currently climbing – is to head down. That is extremely hard for any ambitious, hard working person, organism, organization, or AI.
At the moment generative AI is not good at this. At the moment AI and robots are very good at being efficient, answering questions, and going up gradients towards betterment, but not very good at following hunches, asking questions, and wondering. If, when, they do that, that will be good. My hope it that they will help us humans hill-find better.